All published articles of this journal are available on ScienceDirect.
Social Networking Service and Depression: Evidence from China
Abstract
Background:
The rapid development of Social Networking Service (SNS) all over the world has changed everyone's life, of course, China is no exception. At the same time, the possible depression caused by SNS has attracted the attention of academia, but there is little research on the impact of age heterogeneity, especially in China.
Objectives:
The aim of this study was to further explore the effects of SNS and some factors on depression and to find the differences in these relationships between different age groups.
Methods:
Based on the data of the tracking interview of China Family Panel Studies (CFPS) in 2018 (N= 8,666), this study analyzed the relationship between SNS and depression in China and considered the heterogeneous influence in different age groups and other factors (gender, living in urban or rural, household registration, income, cognitive ability, years of education, health, relationship status, CPC membership, religion, social status, popularity). The theoretical basis of age stages is from Erikson’s Eight Stages of Development.
Results:
(1) The multiple regression analysis revealed that the depression was positively correlated with SNS dependence, on the whole. However, this effect was not always significant in every age group, and it was most positive at the ages of 16-18. (2) The depression was negatively correlated with health and popularity at all levels of age. (3) The depression appeared obviously gender difference, and it was intensifying by increasing age. (4) For the whole sample, the effects of income, cognitive ability, relationship status and social status on depression were significant, however, the situation was different for each individual age group in this article. (5) The mitigate of religious beliefs on depression was significant only at ages 41-65, and the coefficient was unstable. (6) The effects of years of education, living in urban or rural, household registration, CPC membership on depression were not significant in this study.
Conclusion:
The impact of SNS on depression was more significant among young people, especially adolescents. A bad situation of health or popularity could increase the risk of depression. Females were more prone to depression. There were different influences of income, cognitive ability, social status, relationship status and religion on depression in different age groups.
1. INTRODUCTION
In the past two decades, from SixDegrees (which is one of the first social networking websites established in 1997 [1]) to Facebook, we are all the witnesses of the booming development of Social Networking Service (SNS). There were 2.41 billion monthly active users on Facebook, as of June 30, 2019 [2], it was about one-third of the population of the world [3]. In China, the most popular SNS was WeChat which had 1.11 billion active users, as of July 31, 2019 [4]. Recently, however, many researchers found that SNS has brought not only great convenience to our lives but also some problems, such as privacy [5, 6], addiction [7, 8], cyberbullying [9, 10] and mental health [11, 12]. Depression is one of these issues, and it was thought to cause many hazards, such as the risk of suicide and the decline of quality of life [13, 14]. This study focuses on the effect of SNS on depression.
1.1. Literature Review
Published studies on the relationship between SNS and depression have a sundry conclusion. Thomée et al. found that there was a positive relationship between internet communications and symptoms of depression [15]. Wang et al. reported that the time and frequency of SNS use could increase the level of negative emotion and depression [16]. McDougall et al. found that the hours of SNS use per day was associated with depression [17]. Moreover, there are many other studies that indicate that SNS could increase the risk of depression [18-21]. Some articles hold that this effect possibly from the changes in self-esteem, the decrease in physical activity [22], the sense of self-inferiority caused by envy [23], the feeling of “time wasted” by doing something meaningless using SNS [24], the sense of loss owing to the lack of attention [25] and the feeling of information overload [26].
Other studies, however, expressed different opinions. Morgan and Cotten found that the hours of using Internet communication was negatively associated with depressive symptoms [27]. Shaw and Gant argued that chatting with another person online could decrease loneliness and depression significantly [28], and Bessière et al. found that communication with friends and family was negatively correlated with depression [29]. Tandoc et al. found that the effect of Facebook on depression is mediated by Facebook envy, when Facebook envy is controlled for, Facebook use has a negative effect on depression actually [30], and Yan et al. reported that SNS use can reduce the depression level of adolescents significantly, but online social capital could mediate this effect completely [31]. Moreover, some studies hold that there was no significant relationship between SNS and depression [32, 33].
In addition, there are some factors that could affect the relationship between SNS and depression. Some authors indicated that young age could increase the risk of mental problems when people use the internet [34, 35], and SNS use was significantly negatively correlated with depression for older men [36]. Moreover, gender [37], rural-urban [38], income [39], cognitive ability [40], education [41], health [42], relationship status [43], religion [44], social status [45], popularity [46] were considered the influence factors of depressive symptoms. Furthermore, household registration and CPC membership are important demographic variables in China [47], and some studies found that CPC members have a higher sense of happiness [48, 49].
Overall, the relationship between SNS and depression is complex. Although so many studies have been carried out, there were still some limitations. For example, most of the previous studies only focused on unique platform such as Facebook [32, 50], Instagram [51], and the age groups of interviewees were limited [52, 53], or considered the age factor, but no the heterogeneous and the nonlinear analysis [34]. Moreover, there is as yet no research using a large, nationally representative sample of different age groups of China. Compared with previous research, this study aimed to examine a broader range relationship between SNS and depression, and considered about the heterogeneous and the nonlinear factors of age, furthermore, found the effects of SNS and some control variables on depression in different age groups.
2. MATERIALS AND METHODS
2.1. Data
This study uses data from the tracking interview of China Family Panel Studies (CFPS) in 2018 (CFPS 2018). CFPS is a national representative longitudinal survey, using the implicit stratification, multi-stage sampling and probability proportional to size (PPS) methods, and the data is available for the public. CFPS 2018 selected samples from 31 provinces of China, excepting Tibet, Hong Kong, Macao and Taiwan, the original data included 32,669 personal samples and 14,241 family samples. Through the data cleaning and matching, 8,666 samples were used by this study.
2.2. Variables
CES-D (M=13.14, SD=3.53). In CFPS 2018, the Center for Epidemiologic Studies Depression Scale (CES-D) was used to measure the individual depression level. In order to improve the response rate, CFPS 2018 picked 8 questions out of the 20, each of which has four options, corresponding to 1, 2, 3 and 4 points, respectively (APPENDIX). Among them, two questions are reverse scoring. The total score range is 8-32 points. The higher the score, the more serious the depression.
SNS dependence (M=0.68, SD=0.70). This variable is calculated from two questions in CFPS 2018. The first is “How many hours do you normally spend online per week? (0-168 hour(s))”. The second is “How important is social to you when you use the Internet?”, it has five options, from 1 to 5, 1 means very unimportant and 5 means very important. I divided the value of the first question by 7 to get the average daily online time, and divided the second question by 5 as the weight. Via multiplying the average daily online time and the weight, we got the value formed by combining subjective and objective indicators, from 0 to 24. In order to make the variable clearer, I divide it by 24, then multiply by 10, to transform it into a variable from 0 to 10, in this way, we got the SNS dependence. The larger the value, the participant was more dependent on SNS.
Household registration. In China, the household registration system as a special method of population management has made numerous important impacts on everyone. For a long time, Chinese people have been divided into 2 categories by household registration system, one is resident with agricultural household registration, the other is resident with non-agricultural household registration. There were great differences in the aspects of medical treatment, education, housing, welfare and others between the two kinds of household registration. Not only that, but the cross-regional labor flow was also difficult, due to the household registration system. Thus, the household registration is used as a control variable in this study, moreover, the samples with agricultural household registration are defined as 0 (n=5526, 63.77%), and the non-agricultural are defined as 1 (n=3140, 36.23%).
Cognitive ability (M=6.06, SD=1.96). CFPS 2018 conducted the word test and math test for interviewees, which every kind of test included 34 questions, and set different starting points of questions based on their educational background. Using the score of these two variables, this study generated a proxy variable of cognitive ability, and limited its value from 0 to 10. The larger the value, the higher the cognitive ability.
Whether or not the interviewee is a member of the Communist Party of China (CPC). As well known, the political system of China is multiparty cooperation and political consultation under the leadership of the CPC, and the number of CPC members has reached 90.594 million, as of December 31, 2018 [54]. In China, the title of CPC member has a special significance, so this study took it as a factor that may affect the depression, and defined CPC member as 1 (n=1028, 11.86%), otherwise, as 0 (n=7638, 88.14%).
Other variables. In addition to the above variables, the following variables are included in the model: gender (1=male (n=4414, 50.93%), 0=female (n=4252, 49.07%)); age (from 16 to 86. M=38.66, SD=13.78); square of age divided by 100 (M=16.84, SD=11.34); urban or rural (1=urban (n=5106, 58.92%), 0=rural (n=3560, 41.08%)); the natural logarithm of family annual income (M=11.24, SD=0.88); years of education (M=10.00, SD=3.80); self-rated health (from 1 to 5, the higher the value, the healthier the interviewee thought himself. M=3.16, SD=1.10); relationship status (1=married or cohabiting (n=6395, 73.79%), 0=otherwise (n=2271, 26.21%)); religion (1=the member of any religion (n=209, 2.41%), 0=otherwise (n=8457, 97.59%)); self-rated social status (from 1 to 5, the higher the value, the higher social status the interviewee thought himself. M=2.99, SD=0.93); self-rated popularity (from 0 to 10, the higher the value, the more popular the interviewee thought himself. M=7.12, SD=1.70).
2.3. Methods
In this study, the mean value, standard deviation, or the percentages of the variables were statistically described. Stata, version 15.1, was used.
Moreover, the effects of SNS dependence and the above-mentioned control variables on depression were analyzed in the whole sample by multiple regression, using the method of ordinary least squares (OLS) (Table 1). For the whole sample, the OLS model this paper used as following Equation (1):
In the Equation (1),
Furthermore, this study analyzed the effects of SNS dependence and the above-mentioned control variables (excepting the age and the square of age divided by 100) on depression in different age groups, in the same way (Tables 2-5). For different age groups, the OLS model this paper used as following Equation (2):
In the Equation (2), the difference from Equation (1) is the
3. RESULTS
3.1. Multiple Regression Analysis for Variable CES-D
3.1.1. Whole Sample
Table 1 shows the results of multiple regression for the whole sample (Equation 1) using OLS. In general, the CES-D was positively correlated with SNS dependence and age. However, the CES-D was negatively correlated with the square of age divided by 100, it meant that the correlation between CES-D and age was not a single trend of positive or negative, it was closer to inverse U-shape (Fig. 1). Moreover, Table 1 shows that the coefficient of gender variable was negative, it meant that women were more vulnerable to depression. Furthermore, the CES-D was negatively correlated with the natural logarithm of family annual income, cognitive ability, health, social status, and popularity. In addition, people who were married or cohabiting were more likely to have lower levels of CES-D.
Variable | β | SE | t | P>|t| | Sig |
---|---|---|---|---|---|
SNS dependence | 0.147 | 0.052 | 2.84 | 0.005 | ** |
gender | -0.396 | 0.070 | -5.64 | 0.000 | *** |
age | 0.124 | 0.017 | 7.39 | 0.000 | *** |
(age^2)/100 | -0.164 | 0.019 | -8.68 | 0.000 | *** |
urban | -0.128 | 0.084 | -1.54 | 0.124 | - |
household registration | -0.156 | 0.091 | -1.71 | 0.087 | - |
LNincome | -0.333 | 0.045 | -7.47 | 0.000 | *** |
cognitive ability | -0.206 | 0.025 | -8.17 | 0.000 | *** |
EDUyear | -0.024 | 0.013 | -1.76 | 0.078 | - |
health | -0.941 | 0.034 | -27.75 | 0.000 | *** |
relationship status | -0.787 | 0.114 | -6.92 | 0.000 | *** |
CPC membership | 0.159 | 0.115 | 1.39 | 0.165 | - |
religion | -0.360 | 0.226 | -1.59 | 0.111 | - |
social status | -0.282 | 0.039 | -7.22 | 0.000 | *** |
popularity | -0.220 | 0.021 | -10.33 | 0.000 | *** |
*** p<0.001, ** p<0.01, * p<0.05.
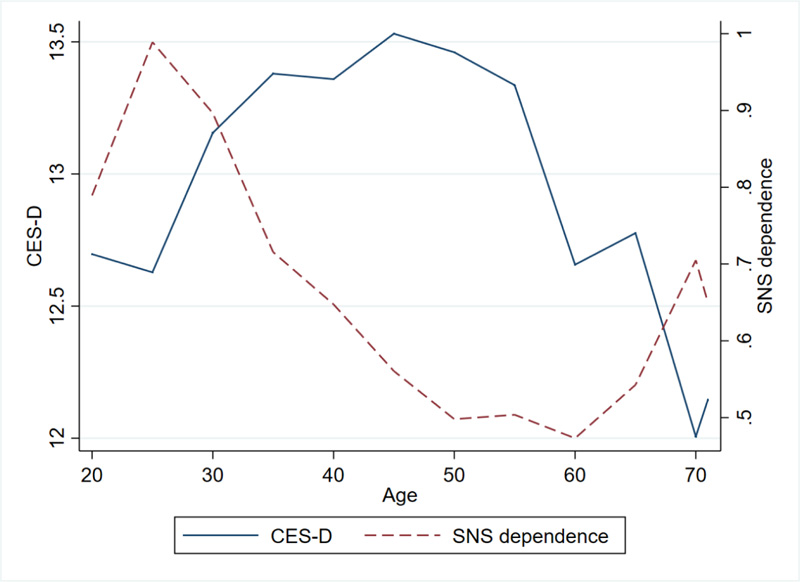
3.1.2. Different Age Groups
In this study, the theoretical basis of age stages is from Erikson’s Eight Stages of Development. It focuses on psychosocial development, and divided life into eight stages based on the personality development [55], they are infancy (0-1.5 years old); early childhood (1.5-3 years old); pre-school age (3-5 years old); school age (5-12 years old); adolescence (12-18 years old); young adult (18-40 years old); adulthood (40-65 years old); old age (more than 65 years old). As McAdams pointed out, Erikson’s theory is trying to understand and explain the entire human life [56].
Many articles indicated that there was a significant relationship between personality and depression [57], and SNS is the product of social development. Thus, it is more suitable for this article than other theories, such as Freud's Psychosexual Developmental Theory. Owing to the age of samples of the data were from 16 to 86 years old, there were no samples belonging to the four age groups in Erikson’s Eight Stages of Development before 16 years old, the sample was divided into four parts by age, they are 16 to 18 years old, 19 to 40 years old, 41 to 65 years old and 66 to 86 years old.
Tables 2-5 show the results of multiple regression in four age groups (Equation 2) using OLS, respectively. The CES-D was positively correlated with SNS dependence at ages 16-18 and 19-40, moreover, the correlation of the former was more positive, but this effect was insignificant in other age groups. However, the CES-D was negatively correlated with health and popularity at all levels of age, and the effects are close. Meanwhile, females might be more likely to have depression above age 18, and this effect was growing with age. In addition, the CES-D was negatively correlated with the natural logarithm of family annual income, cognitive ability and social status at ages 19-40 and 41-65. Furthermore, at ages 41-65, the effects of income and social status on CES-D was more negative, but the effect of cognitive ability on CES-D was less negative than 19-40. Moreover, the CES-D was negatively correlated with the relationship status at ages 41-65 and 66-86, it meant that a stable partner (married or cohabiting) could reduce the level of depression, and this effect was more significant in the 41-65 age group. Besides, religious beliefs might relieve depression, this effect was significant at ages 41-65.
Variable | β | SE | t | P>|t| | Sig |
---|---|---|---|---|---|
SNS dependence | 0.388 | 0.135 | 2.86 | 0.004 | ** |
gender | -0.313 | 0.232 | -1.35 | 0.178 | - |
urban | 0.402 | 0.274 | 1.47 | 0.142 | - |
household registration | -0.339 | 0.320 | -1.06 | 0.290 | - |
LNincome | 0.086 | 0.147 | 0.59 | 0.557 | - |
cognitive ability | -0.128 | 0.081 | -1.58 | 0.115 | - |
EDUyear | -0.149 | 0.081 | -1.83 | 0.068 | - |
health | -0.925 | 0.129 | -7.18 | 0.000 | *** |
relationship status | 0.572 | 1.733 | 0.33 | 0.741 | - |
CPC membership | -1.342 | 0.943 | -1.42 | 0.155 | - |
religion | -0.015 | 0.938 | -0.02 | 0.987 | - |
social status | -0.114 | 0.129 | -0.88 | 0.378 | - |
popularity | -0.229 | 0.072 | -3.18 | 0.002 | ** |
*** p<0.001, ** p<0.01, * p<0.05.
Variable | β | SE | t | P>|t| | Sig |
---|---|---|---|---|---|
SNS dependence | 0.171 | 0.068 | 2.52 | 0.012 | * |
gender | -0.211 | 0.097 | -2.17 | 0.030 | * |
urban | -0.130 | 0.112 | -1.16 | 0.247 | - |
household registration | -0.169 | 0.125 | -1.35 | 0.177 | - |
LNincome | -0.198 | 0.064 | -3.12 | 0.002 | ** |
cognitive ability | -0.212 | 0.035 | -6.01 | 0.000 | *** |
EDUyear | -0.008 | 0.020 | -0.38 | 0.701 | - |
health | -0.949 | 0.048 | -19.77 | 0.000 | *** |
relationship status | -0.184 | 0.113 | -1.63 | 0.102 | - |
CPC membership | 0.143 | 0.168 | 0.85 | 0.395 | - |
religion | 0.344 | 0.358 | 0.96 | 0.338 | - |
social status | -0.266 | 0.057 | -4.69 | 0.000 | *** |
popularity | -0.234 | 0.031 | -7.55 | 0.000 | *** |
*** p<0.001, ** p<0.01, * p<0.05.
Variable | β | SE | t | P>|t| | Sig |
---|---|---|---|---|---|
SNS dependence | 0.004 | 0.103 | 0.04 | 0.972 | - |
gender | -0.640 | 0.118 | -5.45 | 0.000 | *** |
urban | -0.274 | 0.142 | -1.93 | 0.054 | - |
household registration | -0.169 | 0.149 | -1.14 | 0.255 | - |
LNincome | -0.555 | 0.072 | -7.70 | 0.000 | *** |
cognitive ability | -0.167 | 0.043 | -3.88 | 0.000 | *** |
EDUyear | -0.029 | 0.022 | -1.29 | 0.198 | - |
health | -0.899 | 0.052 | -17.12 | 0.000 | *** |
relationship status | -1.538 | 0.226 | -6.81 | 0.000 | *** |
CPC membership | 0.294 | 0.174 | 1.69 | 0.091 | - |
religion | -0.873 | 0.328 | -2.66 | 0.008 | ** |
social status | -0.337 | 0.062 | -5.47 | 0.000 | *** |
popularity | -0.209 | 0.033 | -6.33 | 0.000 | *** |
*** p<0.001, ** p<0.01, * p<0.05.
Variable | β | SE | t | P>|t| | Sig |
---|---|---|---|---|---|
SNS dependence | 0.154 | 0.253 | 0.61 | 0.544 | - |
gender | -0.864 | 0.431 | -2.00 | 0.046 | * |
urban | -0.702 | 0.703 | -1.00 | 0.319 | - |
household registration | -0.647 | 0.705 | -0.92 | 0.360 | - |
LNincome | -0.051 | 0.243 | -0.21 | 0.835 | - |
cognitive ability | -0.159 | 0.157 | -1.01 | 0.314 | - |
EDUyear | -0.027 | 0.067 | -0.40 | 0.689 | - |
health | -0.972 | 0.208 | -4.67 | 0.000 | *** |
relationship status | -1.267 | 0.574 | -2.21 | 0.028 | * |
CPC membership | -0.575 | 0.456 | -1.26 | 0.208 | - |
religion | -0.864 | 0.962 | -0.90 | 0.370 | - |
social status | -0.265 | 0.219 | -1.21 | 0.227 | - |
popularity | -0.277 | 0.136 | -2.04 | 0.043 | * |
*** p<0.001, ** p<0.01, * p<0.05.
4. DISCUSSION
4.1. The Age Heterogeneity Analysis
4.1.1. SNS and Depression
The results of this study showed that the CES-D was positively correlated with SNS dependence in the whole sample, but when we consider the heterogeneity in different age groups, this effect was not significant always, it was most positive at the ages 16-18. In addition to the factors mentioned in the introduction [22-25], the heterogeneous effects of SNS dependence on CES-D in different age groups could be explained by such reasons as follows:
First, the middle-age is a stable period of human psychology, before this, young people are more likely to have fluctuations in psychology, and many mental illnesses emerged during adolescence [58]. As the research has shown that adult personality trait development is continuous through at least age 50 [59]. Moreover, personality was considered a significant factor influencing depression, and this influence could work through a variety of pathways [57].
Second, owing to the various types of things from home and work, the middle-age always has more possible factors that may influence the mood [60] and have less time to use SNS Fig. (1), compared to the younger and older people. Thus, the effect of SNS might be weaker for the middle-age.
Third, considering to the obstructions which limit seniors’ SNS use, such as worry about the privacy and security of internet, impairments or disabilities of vision and memory, lack of computer literacy [61, 62], the author presumed that they may prefer to use the simple functions like communication, rather than the advanced actions such as upvote and comment, it might be a factor attenuated the impact of SNS on depression.
4.1.2. Other Variables and Depression
First, the effect of health and popularity on depression seems stable at all the age groups in this study. Alpass and Neville found that self-reported health was associated with depression, the poorer the health, the more serious the depression, but there was not a significant relationship between long-term disability/illness and depression [42]. Thus, the effect of health on depression might from self-feeling, rather than a realistic situation. Nangle et al. indicated that popularity was significantly correlated with friendship, and friendship influenced depression via loneliness [46].
Second, depression appeared obviously gender difference, and it was intensifying by increasing age. For a long time, many studies found that women are about twice as likely as men to get depression [63, 64]. Numerous scholars indicated sundry reasons of this difference, Hyde et al. proposed the ABC model, explained the gender difference from the affective, biological, cognitive factors, respectively, and indicated that adolescence is an important period for this difference [65] Salk et al. indicated that the peak of gender difference appears in adolescence [66], their conclusion is different from this study, that might cause by the difference of culture, society or nation [67], as the research based on the Chinese data from Zhou et al. also found that the prevalence differs between women and men was increased with age [39].
Third, for the whole sample, the effects of income, cognitive ability, relationship status and social status on depression were significant, however, the situation was different for each individual age group in this article. In fact, there are many studies about the relationships between the four of them and depression [39, 40, 68], as for the age heterogeneity, Schaakxs et al. indicated that the effects of income and relationship status on depression were highest in older age [69], and Beaujean et al. indicated that the relationship between depression and cognitive ability develops in the young age (childhood/adolescence) [70]. However, this paper came to the different conclusions, the effects of income, relationship status and social status on depression were most influential at the ages 41-65, for the cognitive ability, it was at ages 19-40. According to Brockmann, the happiness of middle-aged person mostly from the job and marriage [71], they have a direct impact on income, relationship status and social status, thus, this can be regarded as the evidence of the results of this article. Many authors found that cognitive ability could predict job performance [72, 73], in the author's view, this feeling of work smoothly might make the mood better and reduce depression.
Fourth, the mitigation of religious beliefs on depression was significant only at ages 41-65, and the coefficient was unstable. Koenig indicated that most of the studies found that religious beliefs could reduce the risk of depressive symptoms [74]. However, Brown and Tierney got a contradictory result in China, they found that the religiously-active men got the lower happiness, and they attributed this result to the history of China, such as the Cultural Revolution [75], moreover, Li et al. thought that the phenomenon was the result of the prevalence of Confucianism in China [76]. The result of this study could be regarded as new evidence for related research, this might mean the Chinese people's attitude towards religion was undergoing changes.
Fifth, the effects of years of education, living in urban or rural, household registration, CPC membership on depression were not significant in this study. However, many studies found that education could reduce depression [39, 68], Mirowsky and Ross indicated that education could increase the possibility of employment and marriage, the income, the marital relations, and reduces the depression [77]. In fact, some authors found that the place of residence [78], household registration [79, 80], CPC membership [80] have similar effects with education in China. Thus, the effects of the four of them might work through income, social status and relationship status, and this could the reason why they were not significant.
Overall, as pointed out above, this paper has contributed to the study of the relationship between SNS and depression, explored the effects of some factors on depression, and analyzed the heterogeneous effects of age, through empirical analysis and comparison with previous research. Based on the conclusions of this article, for SNS users, they can adjust the usage style according to their own conditions, for a better psychological state. Moreover, for the SNS developers, they can adjust the operation mode and content for different user groups, for the better user experience and health. For example, considering that adolescents are the most affected by SNS, depression warnings can be given to this age group of users.
5. LIMITATION AND FUTURE DIRECTION
Despite the strengths, there were still several limitations in this study. Initially, there are huge differences between every social networking site or application, even in the same one, the users would get the disparate information. What's more, everyone’s character is different, it could be the influence factor between SNS and depression. Finally, the surrounding elements of historical, cultural, and natural environments might be one kind of impact. Moreover, the influence mechanisms of the control variables mentioned above on depression were still not completely clear. Therefore, the follow-up researches need more meticulous consideration in these aspects.
CONCLUSION
In conclusion, this study showed that SNS dependence has a positive effect on depression, and analyzed this positive effect of the heterogeneity in age. In addition, this study found the effects of health and popularity on depression seem stable at all the age; there was a gender difference of depression, and it was intensifying by increasing age; the effects of income, cognitive ability, relationship status, social status and religious beliefs on depression were different between different age groups. Nowadays, with the popularity of social networks, these analyses have great significance. They can help people not only use SNS more reasonably but also relieve the symptoms of depression.
ETHICS APPROVAL AND CONSENT TO PARTICIPATE
Not applicable.
HUMAN AND ANIMAL RIGHTS
No animals/humans were directly involved in this research.
CONSENT FOR PUBLICATION
Not applicable.
AVAILABILITY OF DATA AND MATERIALS
This study uses data from the tracking interview of China Family Panel Studies (CFPS) in 2018 (CFPS 2018). CFPS is implemented by the Institute of Social Science Survey (ISSS) at Peking University. According to the requirements of ISSS, an institution or individual can apply for the data on http://www.isss.pku.edu.cn.
FUNDING
None.
CONFLICTS OF INTEREST
The author declares no conflict of interest, financial or otherwise.
ACKNOWLEDGEMENTS
Declared none.
APPENDIX
CES-D Questionnaire
The following are some feelings or behaviors you may have. Please indicate the frequency of various feelings or behaviors in the past week according to your actual situation.
Q1-Q8 share the following options: 1. Hardly (less than one day) 2. Sometimes (1-2 days) 3. Often (3-4 days) 4. Most of the time (5-7 days).
Q1. I was upset.
Q2. I thought that it was very difficult to do anything.
Q3. I was not sleeping well.
Q4. I was happy.
Q5. I was lonely.
Q6. I lived a happy life.
Q7. I was sad.
Q8. I cannot continue my life.