All published articles of this journal are available on ScienceDirect.
From a Deliberative to an Implementing Mindset a Process-oriented View of the Formation of Academic Entrepreneurial Intention
Abstract
Background:
Previous intention-based research has not considered whether participants are in the motivational or in the actional phase. In turn, this creates a gap of knowledge concerning the cognitive and motivational processes involved in the formation of Entrepreneurial Intention (EI). By applying the Theory of Planned Behavior (TPB), the present study addresses the formation of EI to commercialize research knowledge, focusing on the transition from motivation to implementation in the context of academia.
Methods:
Drawing on cross-sectional data of 490 researchers, segmented regression analysis was conducted to analyze the influence of entrepreneurial engagement on EI-growth. Multi-group Structural Equalization Modeling (SEM) was then used to test the moderation effects of engagement on the relationship between motivational factors and entrepreneurial intention.
Results and Discussion:
The analysis revealed a direct influence of engagement on EI, as well as a threshold of EI-growth per the context of a Rubicon crossing after the initiation of the first gestation action. Our data also show a growing influence of endogenous factors (e.g., attitudes and perceived behavior control) on EI during the venture creation process. The second part of the study contributes by testing the effects of entrepreneurial rewards on TPB-antecedents moderated by engagement.
Conclusion:
Until today, research mostly relied on cross-sectional data to predict and measure the strength of EI in the phase preceding the launch of a new business without considering whether participants are in the motivational or in the actional phase. Our finding highlights the need to shift from focusing entrepreneurship research solely on intentions to now on the process and implementation perspective.
1. INTRODUCTION
Entrepreneurial Intentions (EI) are considered the first step in a venture creation process. Scholars thoroughly examined the motivational factors that explain EI, for example, in the theory of planned behavior. However, previous intention-based research has not considered whether participants are in the motivational phase (i.e., aspiring entrepreneurs) or in the actional phase (i.e., nascent entrepreneurs focused on implementation). Only recently, scholars applied the mindset theory of action phases and found evidence that cognitive processes differ in the motivation and actional phases [1, 2]. In turn, this creates a lack of knowledge concerning the cognitive and motivational processes involved in the formation of EI, also referred to as goal intentions, within the phase preceding the launch of a new business.
Transitioning entrepreneurship research from goal-oriented motivations to an implementation-oriented phase is based upon the mindset theory of action phases (MAP) and the related Rubicon model of action phases [3, 4]. In response to the increasing demand for approaches that reflect the complexity of implementing entrepreneurial action, recent studies [1, 5, 6] applied the Rubicon model, which is associated with two different types of intentions: the “goal intention”; and, the “implementation intention” [7]. The formation of goal intention (i.e., a mental representation of the desired outcome) is the first step in a venture creation process, followed by implementation intentions in the pre-actional and actional phases. Entrepreneurship research recently confirmed the Rubicon model’s assumption that the transition from a motivational to a volitional stage (i.e., the crossing of the Rubicon) is associated with a change in the cognitive mindset [1]. Accordingly, it was reported that individuals in the motivational phase focused exclusively on information relevant to achieving their goal, instead of those individuals in the motivational phase, whose focus was evaluating desirability and feasibility [1]. The authors also noted that the intention construct used in TPB related to the construct of goal intention in the motivational phase of the Rubicon model.
However, previous intention-based research has not considered whether participants are in the motivational phase or in the actional phase. In turn, this creates a lack of knowledge concerning the cognitive and motivational processes involved in the formation of goal intentions, highlighting prior research’s lack of controlling for whether individuals implemented gestation actions were derived from a deliberative or implemental mindset. Against this background, three research questions become evident. First, there are insufficient data on the role of Entrepreneurial Engagement (ENG) in the motivational processes behind entrepreneurial (goal-) intention. Secondly, future research must integrate and examine engagement as a construct in entrepreneurship research using established explanatory models for entrepreneurial intentions [8, 9]. Thirdly, there is a lack of knowledge regarding the effect of engagement on the relationship between established endogenous and exogenous factors (e.g. entrepreneurial rewards) on entrepreneurial intention. To fill these voids, the aim of the present study is twofold.
Based on the TPB and MAP, the first part of this study investigates whether there is a positive effect of entrepreneurial engagement, which extended beyond the antecedents of TPB to explain entrepreneurial intention. Furthermore, we investigate if intention increased linearly during the entrepreneurial process or if growth peaked for EI per the context of a Rubicon crossing. We also test whether ENG moderates the relationship between TPB predictors (e.g., social norms, attitudes, and perceived behavioral control) and EI. Part one constructs an improved picture of EI before progressing into part two of the study.
The second part of this study addresses the lack of understanding concerning the effects of framework conditions on the entrepreneurial decision-making process. The study analyzes the institutional determinants of EI by combining motivational aspects of TPB and environmental factors to predict EI. Part two commenced with an institutional perspective [10] by addressing environmental factors, namely entrepreneurial rewards that encourage entrepreneurship among academics to predict EI [11-13].
Overall, this study investigated the extent to which engagement alters the motivational effect of exogenous factors on TPB predictors and goal intention in the phase preceding the launch of a new business. We draw on data from academic entrepreneurship. Academic research commercialization is crucial for transmitting knowledge from research to society [14]. Experts considered academic entrepreneurship as all commercialization activities (e.g. spin-off activities) outside the regular university tasks of basic research and teaching [15]. Academic spin-offs are defined as “companies founded by individuals from the scientific community, including people with substantial research experience such as professors, assistants, researchers, and doctoral students and based on a core technology that is transferred from the parent organization” [11]. Therefore research on academic entrepreneurship is an example of the pivotal role of intention-based research and of the application of TPB [16, 17].
The present research used a cross-sectional data set of academic researchers from Switzerland and employed Structural Equation Modelling. In doing so, this paper yielded interrelated contributions to the literature and highlighted the essential role of action-related approaches for studying entrepreneurial intention.
2. LITERATURE REVIEW
2.1. Entrepreneurial Intention
Entrepreneurial intentions are considered to be the “single best” predictor of entrepreneurial behavior [18]. Following this statement, the use of EI as a surrogate for action in empirical research has been established within entrepreneurship research. Many motivational theories are based on the concept of intention and their antecedents to predict subsequent actions [8, 18-20]. While these theories primarily capture future action as outcome variables, the majority of research has focused exclusively on predicting EI rather than performance [21].
In particular, the TPB [8], an extension of Ajzen’s Theory of Reasoned Action [22], comprised most of the research on intention within the field of entrepreneurship [21]. TPB is a psychological theory and posits the process of EI formation as determined by three independent constructs: positive or desirable assessment of a certain behavior (shorted as ATT); the perceived social norm (shorted as SN); and, the perceived feasibility of performing or not performing a certain behavior (shorted as PBC). The effects of ATT, SN, and PBC are meditated by EI on subsequent behaviors [23].
In academic entrepreneurship research, TPB is utilized to investigate the motivational influence of personal and contextual factors on entrepreneurial goal-setting [24-26]. Based on TPB, Feola et al. [11], investigated how entrepreneurial universities influence EI among scientists1. To assess for the success of certain framework conditions in entrepreneurship, the scholars considered the strength of EI as a sufficient predictor of the probability of taking action.
While a positive relationship between intention and subsequent behavior has been corroborated in studies in the field of entrepreneurship [27], the predictive value remains limited. For example, by using longitudinal data, Kautonen et al. [27], demonstrated that (goal-) intention elucidated about 30% of the variance in subsequent entrepreneurial actions. Furthermore, the authors noted that only 37% of the respondents who reported positive EI in the first wave took subsequent action, in the second wave.
Earlier research showed that EI strength is greater among those who are currently involved in implementing a business project [28], however, there is a lack of empirical studies that explicitly control whether individuals started to implement their entrepreneurial goals. As meta-analytically shown, the effect size of manipulating goal intention strength is rather limited [29]. Therefore, it is worth questioning whether goal intentions increase linearly during the entire business process or whether EI-growth reaches a plateau after entrepreneurship has been defined as a goal.
In line with this, van Gelderen et al. [6] recently stated that the strength of EI is not a sufficient predictor of subsequent behavior. By drawing on MAP, the authors found a moderated mediation, in which implementation intention mediated the effect of goal intention on taking entrepreneurial actions.
2.2. From a Deliberative to an Implementing Mindset
Based on the Rubicon model [4, 30], decision-making and action implementation are processed in two different phases. First, in the motivation phase, goals and alternatives are weighed against each other in terms of feasibility and desirability [30]. Therefore, costs (e.g., in terms of effort) and benefits are compared rationally to make final (goal-) decisions [3]. This process leads to the formation of goal-intention, which is a construct comparable to the motivational TPB concept of intention. After goal-setting, individuals set out to implement and achieve the goals [30].
Second, in a volitional phase, implementation intentions are formed, related to the question of 'How can I achieve my goal' [31]. Social psychologists refer to the transition from a deliberative to an implementing mindset, as the crossing of the Rubicon. In this stage, an implemental mindset automatically facilitates the initiation of goal-oriented action by drawing the decision-maker's attention to available implementation strategies for achieving goals. In contrast to the motivation phase, individuals in the volitional phase are focused on how to achieve desired goals, whereby both feasibility and desirability of goals are perceived in a rather partial and overly optimistic way [4]. Furthermore, information that challenges the usefulness of goals or raises any lingering doubts is not well processed. New information is only perceived if it is relevant to the achievement of goals (i.e., closed-mindedness) so that the attention is guided towards available resources for deploying the decision.
3. DEVELOPING HYPOTHESES
3.1. Entrepreneurial Engagement Positively Predicts EI
Delanoë Gueguen and Fayolle [1] recently proposed and empirically demonstrated that entrepreneurial engagement (measured by the sum of gestation action2) could be operationalized to determine whether potential founders crossed the Rubicon. Based on longitudinal data, the authors found evidence of a positive influence on both ENG and goal intention, on subsequent entrepreneurial action, and a moderating effect of ENG on the relationship between intention and behavior. As theorized, by applying the Johnson-Neyman technique (moderation technique), the positive effect of intention on behavior disappeared beyond the ENG threshold of three gestation actions. This finding suggests that at a certain point in the entrepreneurial process, EI was less powerful for predicting action. Delanoë Gueguen and Fayolle [1] interpreted this moment as representing the presence of a Rubicon crossing, and argued that the initial gestation actions represent a way to generate goal-oriented information in the motivation phase. This result contrasts with the assumption that the transition of a Rubicon is theoretically considered to be the moment when individuals actively engage in implementation [30]. Depending on the context and the topic of entrepreneurial projects (e.g., cultural versus technological entrepreneurship), both the order and the content of gestation action differ.
As mentioned above, intention strength is considered a sufficient predictor for the achievement of goals (e.g., in the context of TPB). We argue that a linear growth of EI, especially concerning goal-intention, along the entrepreneurial process can be questioned. EI-growth is likely to reach a threshold where the rate of increase slows after leaving the motivation phase. An example of a possible EI threshold was demonstrated in entrepreneurship education. A meta-analysis [34] of 73 studies revealed only a weak correlation between education and EI. If, however, the authors controlled for pre-education intention strength, then the correlation was insignificant. In entrepreneurship education research, this effect has been discussed previously as a result of a bias in self-selection [35], indicating that EIs are unlikely to grow if people with a high level of interest enroll in such a course [34]. For example, Fayolle and Gailly [36] showed that while education has a positive effect on AT and PBC, among all participants, it does not positively influence the EI of participants with previous entrepreneurial experience. Their results suggested EI-growth peaked as soon as goals were defined.
In line with Delanoë Gueguen and Fayolle [1], Mwangi and Rotich [28] recently showed that ENG elucidated variance in EI. Yet, a gap remains concerning the empirical knowledge of EI strengths continued growth after the transition from motivational to volitional phase. However, psychologists argue that detected difficulties and obstacles in achieving goals during implementation increase the overall motivation and goal-commitment, as it supports the process of imitated goal pursuits by mobilizing effort [37]. In turn, the long-term goal is constantly updated by carrying out micro-tasks for implementation. Thus, an increase in goal intention during implementation is likely, as the person becomes more committed to the goal by continually updating the desirability and feasibility of the long-term goal.
Drawing on prior research, we assume ENG to predict EI at the beginning of the entrepreneurial process (motivational phase), and we further assume a significant difference of EI-growth (i.e., threshold) after initiating the first gestation action. Considering the theoretical assumptions of MAP and the results of prior research, we hypothesize that:
H1a) Entrepreneurial engagement positively predicts EI.
H1b) A threshold of EI-growth can be determined in relation to ENG, so that ENG has a greater influence on EI before the threshold than after the threshold.
3.2. TPB-Antecedents in the Motivation and Volition Phase
In addition to the unique and significant relationship between entrepreneurial engagement and EI, we expect an interactive effect of engagement and TPB to emerge in the model. This prediction is supported by the aforementioned concept of the Rubicon Model, and the related different cognitive processes involved before and after crossing the Rubicon [30]. We assume that the influence of TPB-antecedents on EI, before and after engaging in implementation varies. An implementing mindset promotes a positive assessment of the goal (i.e., desirability) and encourages an optimistic assessment of its achievability [30]. Following our prior argumentation, while individuals with an implementation mindset are centered on specific information related to the successful implementation of their entrepreneurial projects [1], the TPB-antecedents (ATT, SN, PBC) theoretical respond to the motivation phase and thus to the prediction of goal intention.
Previous academic entrepreneurship research yielded mixed results regarding the strength of the influence of TPB-antecedents on EI [24, 25]. For example, Miranda et al. [24] found no significant effect of SN on EI, while other scholars documented a strong effect of SN on EI [11]. Some scholars found evidence that ATT has the greatest effect on EI [24, 26, 38], while other suggested PBC to have the greatest influence on EI [11]. However, most of these studies did not control whether participants were currently involved in the motivational or implementation phase.
Given the mixed results regarding the effects of TPB, and the antecedents of EI, we assume entrepreneurial engagement to moderate the relationship between TPB-antecedents and EI. Following our prior evaluation, individuals with an implementation mindset use different cognitive information processes in relation to the successful implementation of their entrepreneurial projects, in contrast to those individuals with a deliberative mindset [1]. In the motivational phase, new information is assessed more objectively than in the volitional phase [30]. Therefore, nascent entrepreneurs in the implementation phase focus on how to best achieve their chosen goal and perceive both feasibility and desirability, rather partially and optimistically [1]. In contrast to the motivational phase, individuals in the implementation phase display higher levels of self-esteem [39], a lower assessment of their vulnerability to controllable and uncontrollable risks [40] and prefer information that leads to the implementation of goals, with an optimistic view of future performance. Since positive information contributes to justifying difficult and risky goal-decisions like entrepreneurial goals, we argue that the TPB-predictors are more crucial for predicting EI after crossing the Rubicon. Overall, we hypothesize entrepreneurial engagement to moderate the relationship between TPB-antecedents and EI and we assume that the effect of TPB antecedents in explaining EI strength will increase with engaging in implementation.
H1c) ENG has a moderating effect on the relationship between TPB-antecedents and entrepreneurial intentions (the criterion), such that when ENG is low, the relationship is weaker and when ENG is high the relationship is stronger.
3.3. Applying the Interaction Approach on the Context of Academic Entrepreneurship: Entrepreneurial Rewards
Institutions such as universities can guide the process of entrepreneurial decision-making among students [41] and scientists [11, 12, 24] and support their implementation with concrete measures [42]. Institutional theory [10] presents a compelling approach to investigate entrepreneurial behavior in organizations determined by culture and incentives [43]. In particular, Kirby et al. [44], outlined formal factors (e.g., infrastructure and financial support) and informal factors that promote entrepreneurial behavior among university scientists. Similarly, Huyghe and Knockaert [13] empirically analyzed how university culture (e.g., entrepreneurial mission and role models) as well as climate (e.g., financial rewards for commercialization of research) influence entrepreneurial intention among scientists. Based on survey data, the authors found evidence of a unique and direct relationship between university rewards for spin-offs (i.e., start-ups) and EI.
Incentives and rewards are decisive instruments that foster organizational norms in terms of entrepreneurship within universities [45, 46] and influences EI among scientists. In particular, rewards refer to social or financial benefits that influence employees' attitudes towards a particular issue and, in the case of entrepreneurship, increase the likelihood that academics will pursue such goals [47]. As demonstrated by Muscio et al. [48], the existence of monetary incentives embodies the converging signals for researchers to engage in technology transfer activities. For example, Guerrero and Urbano [49] evaluated researchers enrolled in Spanish universities and found evidence for a direct influence of university policies (e.g., including financial rewards) on individual attitudes towards entrepreneurship. Similarly, Miranda et al. [24] obtained comparable results by examining the influence of personal (e.g., self-confidence, business experience) and university-specific entrepreneurial conditions (e.g., business environment) on entrepreneurial intentions mediated by TPB-antecedents amongst a sample of Spanish scientists. Based on previous research [50], we assume that entrepreneurial rewards as part of the institutional climate positively influence the motivation and behavior of institutional members.
It is acknowledged that the institutional environment is a critical element in the formation of EI among academics [44, 51]. Moreover, the entrepreneurial climate within universities, which is positively influenced by cooperation with industry, has a significant influence on ATT of academics. For example, Goethner et al. [25] observed that the climate created by collaboration with industry indirectly influences the EI by affecting the ATT. Similarly, Foo et al. [12] pointed out that scientists with an entrepreneurial background can be stimulated to develop entrepreneurial goals through an encouraging environment. The authors explained that this is influenced by socialization processes within institutions. Also, Obschonka et al. [52] found evidence of socialization effects using data collected from university scientists. When the participants identified with their institution, they tended to adopt attitudes from their social environment, while the institutional environment strongly influenced their entrepreneurial decisions.
Several recent studies have consistently shown that the relationship between contextual factors and EI is mediated by TPB-antecedents such as PBC, SN, and ATT [11, 25]. Therefore, we propose that ATT and SN mediate the relationship between entrepreneurial rewards and entrepreneurial intention. In line with TPB's assumption [8] that contextual factors indirectly influence the EI by altering ATT, SN, and PBC, we propose a mediated relationship. Given the above information, the following hypotheses concerning entrepreneurial rewards were derived:
H2a) ATT mediates the relationship between entrepreneurial rewards and EI.
H2b) SN mediates the relationship between entrepreneurial rewards and EI.
Mwangi and Rotich [28] noted that the application of MAP in entrepreneurship research draws attention to the complex interaction between situational, contextual, and psychological factors in the development process of EI. Based on our prior argumentation, we assume the influence of entrepreneurial rewards on TPB-antecedents to differ before and after engaging in entrepreneurial implementation. Therefore, we argue that entrepreneurial rewards are most important as part of the entrepreneurial climate in university faculties during the motivation phase. In this phase, the advantages and disadvantages of entrepreneurial action are examined to generate goal-intentions (i.e., the so-called readiness for entrepreneurial action). Again, by drawing on MAP, it can be assumed that in the implementation phase, concrete measures, such as formal factors (e.g., business support), will become more important than motivating incentives. Therefore, we hypothesize a moderating effect of ENG on the relationship between entrepreneurial rewards and ATT, respectively SN.
H2c) ENG moderates the relationship between entrepreneurial rewards and ATT.
H2d) ENG moderates the relationship between entrepreneurial rewards and SN.
4. METHODS
4.1. Data Collection
This research is based on cross-sectional data collected in a nationwide, online survey of academics at the seven public Swiss universities of applied sciences (UAS) in 2019. Since 1995, the Swiss UAS expanded its activities in research and development, which is anchored in the legal performance contract [53]. Intensive cooperation with non-institutional actors in the context of practical or business-oriented education and practice-oriented research is seen as a central feature of the profile of UASs [54, 55]. In recent years, the promotion of science-based start-ups has become an integral part of the service spectrum of higher education in Switzerland. Accordingly, many universities and UASs introduced measures to promote the commercialization of research and demonstrate a high entrepreneurial potential [54].
From January to March 2019, 8,905 academics were randomly invited via e-mail to take part in an online survey to examine their entrepreneurial behavior and organizational environment. Participants could choose between three languages (German, English, and French). Only 2,442 academics followed the link to the survey tool and accessed the privacy policy on the first page. In the invitation e-mails, as well as in the introduction to the questionnaire, all participants were informed about the length of the survey and about the background of the questions concerning commercial knowledge transfer. The security of data storage and the maintenance of anonymity in the evaluation of the questionnaire were promised to reduce the tendency of respondents to give socially desirable answers [56]. Participation in the study was voluntary, and responses were anonymous. Beforehand, the questionnaire and the procedure were both tested and optimized using an independent sample population. Participants received three reminder e-mails, each after one week.
4.2. The Sample
The study sample size contained 490 participants. Previously, we removed the respondents from our sample who did not provide any data and those with missing data on gestation actions, and entrepreneurial intention as both variables are key aspects of this study. On average, participants were 44.4 years old (SD = 10.7, range 24-69) and 73.5% (n = 360) of them were male, 69.8% (n = 342) were Swiss citizens, 39.4% (n = 193) stated to hold a PhD as their highest educational qualification. Of the academics responding, 33.3% (n = 163) stated their work as being a professor. About 54.7% (n = 268) of the participants represented the STEM disciplines, which include mathematics, computer science, science, and technology, while the others represented humanities and art, and economics. Further descriptive data are listed in Table 1. The sample corresponds to the demographic distribution in terms of age, nationality, gender, and education of scientists at the Swiss UASs [57].
4.3. Measures
Self-reporting scales employed in this study were successfully applied in previous research and relate to the target behavior of academic entrepreneurship [13, 52, 58]. To test our hypothesis, we drew on previous research to assess entrepreneurial intention as a dependent variable [11, 13, 25, 59]. The questionnaire and data are available upon request from the authors.
- | - | Frequency | Percentage |
---|---|---|---|
Gender | Male | 360 | 73.5 |
Female | 130 | 26.5 | |
Age | Mean (SD) | 44.4 (10.7) | |
Median [Min, Max] | 45.0 [24.0, 69.0] | ||
Discipline | Humanities | 222 | 33.3 |
Natural and Technical Sciences (STEM) | 268 | 66.7 | |
Occupational status | Lecturer / Professor with leadership responsibility | 163 | 33.3 |
Lecturers without leadership responsibility | 123 | 25.1 | |
Research assistant | 204 | 41.6 | |
Employment level | Mean (SD) | 79.5 (24.8) | |
Temporary Employment contract | 163 | 33.3 |
4.3.1. Dependent Variable
Entrepreneurial intention (EI). The responses were measured using a seven-point Likert scale ranging from 1 (Absolutely disagree) to 7 (Strongly agree) to measure EI, (e.g., ‘You have the firm intention of becoming an entrepreneur one day’) based on prior research [1, 60]. Scale reliability, which was measured using Cronbach's α, was found to be 0.86. This was above the generally accepted criterion of 0.70, indicating high reliability for the collected data [61].
4.3.2. Independent Variables
TPB-Social norm (SN) factors were measured using three items on a five-point Likert scale [52], referring to academic colleagues and superiors at the workplace. Participants received the following question: Which of the following people think that you should participate in the development of a business idea to commercialize your research? (i) (work) colleagues; (ii) supervisor or superior; (iii) family and friends. Again, the items were assessed on a five-point Likert scale from 1 (Absolutely disagree) to 5 (Strongly agree) and averaged (α = 0.85).
TPB-Attitudes (ATT) towards academic entrepreneurship refers to the degree to which a person develops a positive or negative assessment towards entrepreneurial behavior. ATT was measured on a five-level bipolar adjective scales. For example, “I consider participation in the development of a business idea to commercialize my research…” Responses included: Item 1: 1= “boring”, 5 = “exciting”; Item 2: 1= “unattractive”, 5 = “attractive”; Item 3: 1 = “uninteresting”, 5 = “interesting”; Item 4: 1= “waste of time”, 5 = “worth investing as much time as possible.” The scale was found to be internally consistent with a scale reliability of Cronbach's α = 0.90.
TPB-Perceived behavioral control (PBC), four items were used on a seven-point Likert scale to measure PBC, with responses ranging from 1 (strongly disagree) to 7 (strongly agree). The three items were (1) “I can control the creation process of a new company,” (2) “I know how to develop an entrepreneurial project,” (3) “I know the necessary practical details to start a company,” and (4) “If I tried to start a firm, I would have a high probability of succeeding.” The resulting scale reliability was Cronbach's α = 0.90.
University rewards for commercialization. Based on Huyghe and Knockaert [13], we created five items to deduce whether the university reward system values academic entrepreneurship. Using a seven-point Likert scale ranging from 1 (strongly disagree) to 7 (strongly agree) all participants were invited to rate the following statement: “Please indicate the activities for which you will receive (social, financial) recognition at your university: (1) Involvement in spin-off/start-up creation, (2) Involvement in patenting and licensing (3) Involvement in consulting/services (4) Providing scientific services for private companies, and (5) Involvement in contract research.” Conducting PCA uncovered the existence of two factors, accounting for 60% of the cumulative variance, we named “entrepreneurial rewards” (Items 1 and 2; Cronbach’s alpha = 0.75) and “rewards on contract-research and consulting” (Items 3, 4, and 5; Cronbach’s α = 0.73). Consequently, we generated summarized measures for the two constructs. In the following analyses, we controlled for “emphasis on contract-research and consulting.”
Entrepreneurial engagement (ENG). To reflect the level of engagement of respondents, we followed the approaches used by prior research [1, 32], which relied on the count of gestational actions reported by individuals. The distribution of the number of gestation actions is displayed in Table 2. The survey included 14 adjusted gestation actions based on lists compiled by Delanoë Gueguen and Fayolle [1] and the US. Panel Study of Entrepreneurial Dynamics (PSED) [33]. Participants were asked: “Which of the following actions have you already undertaken to set up your company?” Respondents were asked to select all activities they had participated in, including: preparation of a business plan, contacts to obtain financing, full-time work on this project, search for public aids, renting and/or equipping a business space, significant investment in the development and/or manufacture of products or services, gathering of information regarding administrative formalities for company creation; meeting with potential clients, registration of patent, name, or trademark, gathering and preparing specific information for potential investors, taking advice from professionals about the implementation of the project, savings to invest in this project, developed and realized prototypes, and already launched products on the market.
4.3.3. Control Variables
Based on findings from academic research on entrepreneurship [16, 17], we selected gender (0 = male, 1 = female), age, occupational status, and highest educational qualification, employment level, and discipline, as control variables to reduce the possibility of alternative explanations for the following results. Prior research showed that male academics had more social contacts with the outside world and fewer systematic barriers than female academics, which explained the greater entrepreneurial activity by men [62-64].
Entrepreneurial Engagement | Frequency | Percentage |
---|---|---|
(0) | 201 | 41.0 |
(1) | 62 | 12.7 |
(2) | 41 | 8.4 |
(3) | 40 | 8.2 |
(4) | 31 | 6.3 |
(5) | 26 | 5.3 |
(6) | 24 | 4.9 |
More than (7) | 65 | 13.3 |
Total | 490 | 100 |
We controlled for employment level to ensure that academics were comparable across all of the involved universities. Furthermore, we controlled for discipline (0 = humanities and art, 1 = STEM) since the research discipline and activities affect entrepreneurial intention [13, 65]. In particular, academics working in applied research and in technical disciplines (e.g., engineering and physics) are more likely to be involved in entrepreneurial activities, while academics in the humanities are more likely to work in consultancy and contract research [43, 59, 63].
4.4. Construct Validity and Reliability
Before testing the hypotheses, exploratory factor analysis (EFA) was used to extract and evaluate the initial construct validity and reliability of the metrics. EFA included examining for items with commonalities, their factor loadings, and Cronbach’s α. As suggested by Hair et al. [66], items with communalities below 0.50, or factor loadings below 0.50 and significant cross-loading were removed. This included the following items: SN (3) family and friends. After adjustments, the item’s commonalities exceeded the threshold of 0.50, and the six factors explained 66% of the total item variance. The items’ factor loadings and labels of extracted factors are reported in Table 3. The measurement items loaded to their respective factors as expected, indicating initial convergent and discriminant validity as factor loadings exceeded 0.50, and cross-loadings were below 0.30. The Cronbach’s alpha coefficients for the measurement items all exceeded 0.70, indicating internal consistency [66].
A confirmatory factor analysis (CFA) was conducted to evaluate the convergent and discriminant validity of the measurement items. Unlike most regression models, the null hypothesis in a SEM is the model perfectly predicts the data; the Chi-square test for the measurement model was significant (X2[120] = 288.7, p < 0.01), indicating a poor fit. However, since the Chi-square value is strongly influenced by sample size, leading to a statistically significant test, but a predictively insignificant model. Hence, alternative fitting indices were used. To further investigate the goodness-of-fit, evidence of model fit was obtained through multiple sources: Chi-square statistic (χ2), assessing the difference between the expected covariance matrix and the observed covariance matrix; Root Mean Square Error of Approximation (RMSEA), which assesses the fit between the covariance matrix of the best fit model and the covariance matrix of the data; and Comparative Fit Index (CFI). The CFI and RMSEA showed a good fit for the measurement model (CFI = 0.96, RMSEA = 0.05) [67] and confirmed a sufficient convergent and discriminatory validity as items loaded significantly on their respective factors and all factor loads exceeded 0.60. Convergent validity can be investigated by calculating the Average Variance Extracted (AVE) using a cut-off of 0.50 [68]. The inspection of the AVE values (Table 3) for all factors suggests acceptable validity was obtained.
Discriminant validity was evaluated by comparing the constructs’ values of the Squared Root of AVE (√AVE) with the correlation of the other constructs [69] (Table 3). A value of √AVE that is higher than the coefficient of the correlation between factors provides evidence of discriminant validity. All factors met the criterion and demonstrated discriminant validity. Second, discriminatory validity was assessed using the heterotrait-monotrait ratio of correlation (HTMT) [70]. HTMT is the average of the heterotrait-hetero method correlation relative to the average of the monotrait-hetero method correlation. If HTMT is below 0.90, a discriminatory validity between two constructs can be assumed. Results show that the HTMT values between the respective constructs appeared to be below 0.90 (highest value of HTMT = 0.61 for the link between Spin-off Rewards and Contract-research Rewards, lowest HTMT = 0.08 for Contract-research Rewards and PBC). The results provide evidence for convergent and discriminant validity.
A methodological problem that must be accounted for is the Common Method Variance (CMV). CMV occurs when a method bias affects all measures equally [71] by systematically distorting participants' responses to surveys (e.g., according to social desirability). To investigate the potential for CMV, all study variables were loaded to one factor to investigate the fit of the CFA model. If the one-factor CFA model fits the data, CMV is considered as responsible for the relationship between the variables [72]. Within this data, a one-factor CFA model did not represent the data well (X2[134] = 2’935, p < 0.001, CFI = 0.38, RMSEA = 0.21), suggesting that the items were not just different aspects of an underlying construct (CMV).
4.5. Analytic Strategy
Hypothesis testing was estimated using Structural Equation Modelling performed with {Lavaan} (Version 0.6-5) [73] in R [74]. SEM examined the relationship between the latent variables and tested the specific hypotheses. Given the sample size, we included only cases with complete data in each analysis. Our data being non-normal and incomplete, we used the Robust Maximum Likelihood (MLR) estimation method [75]. The MLR estimator generates maximum likelihood parameter estimates with standard errors and χ2 test statistics that are robust to non-normality and missing data. Model fit was estimated using several convergent indices: the robust Yuan-Bentler scaled Chi-square test; RMSEA; and CFI. To assess the model fit, we used the following criteria for a satisfactory fit: CFI > 0.90; RMSEA < 0.08, χ2/df < 3.00 [67, 76]. For measurement invariance tests, we used criteria for large samples: p-value of < 0.01 for Δχ2 (scaled difference Chi-square test [77];) and ΔCFI > 0.002, which indicated a lack of measurement invariance [78].
Construct | Item | Factor Loadings |
Cronbach’s Alpha |
Average Variance Extracted (AVE) | √AVE |
---|---|---|---|---|---|
Entrepreneurial Intention (EI) | EI 1 | 0.84 | 0.86 | 0.69 | 0.83 |
EI 2 | 0.81 | ||||
EI 3 | 0.61 | ||||
Social Norms (SN) |
SN 1 | 0.91 | 0.85 | 0.74 | 0.86 |
SN 2 | 0.74 | ||||
SN 3 | 0.47 | ||||
Perceived Behavioral Control (PBC) |
PBC 1 | 0.82 | 0.90 | 0.72 | 0.85 |
PBC 2 | 0.86 | ||||
PBC 3 | 0.90 | ||||
PBC 4 | 0.66 | ||||
Attitudes towards Academic Entrepreneurship (ATT) |
AT 1 | 0.75 | 0.90 | 0.70 | 0.83 |
AT 2 | 0.88 | ||||
AT 3 | 0.84 | ||||
AT 4 | 0.73 | ||||
Entrepreneurial Rewards |
Ent. Rewards 1 | 0.94 | 0.75 | 0.57 | 0.76 |
Ent. Rewards 2 | 0.50 | ||||
Contract-Research Rewards | Cont. Rewards 1 | 0.78 | 0.73 | 0.50 | 0.71 |
Cont. Rewards 2 | 0.56 | ||||
Cont. Rewards 3 | 0.72 |
Segmented regression analysis [79] is used for empirical detection of the EI-breakpoint analysis. A segmented regression (i.e., Brocken-stick-regression), is a method of regression analysis in which the independent variable, namely entrepreneurial engagement, is partitioned into intervals and each interval is assigned a separate line segment. Segmented regression was calculated in {segmented} [80] using entrepreneurial engagement as the independent variable, and EI served as the dependent variable. The algorithm must be supplied with one or more initial guess parameter(s) for the breakpoint(s). We used an initial parameter of ψ0 = 1. In piecewise regression, the relation between the response and the predictor is piecewise linear (i.e., two or more lines are connected at the change point(s)). Empirically determined breakpoints were tested for statistical significance using the Davies test [81]. Using results from the segmented regression, two groups were obtained (pre-breakpoint vs. post-breakpoint). Multi-Group SEM analyses were conducted to determine whether the link between TPB variables and EI was moderated by entrepreneurial engagement.
5. RESULTS
Descriptive statistics and zero-order correlations among variables are listed in Table 4, and the reported correlations are in the expected direction. TPB-antecedents (ATT, SN, and PBC) showed positive correlations with intentions. ENG revealed positive correlations with EI and the TPB constructions. Entrepreneurial rewards correlated with EI, while contract-research rewards did not correlate with EI.
Variable | M | SD | (1) | (2) | (3) | (4) | (5) | (6) |
---|---|---|---|---|---|---|---|---|
(1) Entrepreneurial Intention (EI) | 3.25 | 1.61 | - | - | - | - | - | - |
(2) Attitudes towards academic entrepreneurship (ATT) | 3.75 | 1.02 | 0.44** [0.37,0.51] |
- | - | - | - | - |
(3) Perceived behavioral control (PBC) | 4.42 | 1.35 | 0.41** [0.33, 0.48] |
0.19** [0.11,.28] |
- | - | - | - |
(4) Social Norms (SN) | 3.24 | 1.66 | 0.33** [0.25, 0.41] |
0.24** [0.16, .32] |
0.22** [0.13, 0.30] |
- | - | - |
(5) Entrepreneurial Rewards (ER) | 2.66 | 1.53 | 0.20** [0.11, 0.28] |
0.20** [0.12,0.29] |
0.06 [-0.03,0.15] |
0.32** [0.24,.40] |
- | - |
(6) Contract-research Rewards | 4.23 | 1.47 | 0.07 [-0.02, 0.16] |
0.12** [0.03, 0.21] |
-0.01 [-0.10, 0.08] |
0.23** [0.14, 0.31] |
0.44** [0.37, 0.51] |
- |
(7) Entrepreneurial Engagement | 2.28 | 2.57 | 0.40** [0.32, 0.47] |
0.20** [0.12,0.29] |
0.45** [0.38,0.52] |
0.30** [0.21,0.38] |
0.16** [0.07,.25] |
0.08 [-0.01,0.17] |
5.1. The Empirical Path Model for Predicting Entrepreneurial Intention
We tested our hypotheses on unique main effects as well as moderating effects of ENG in the prediction of EI (hypotheses 1a-c), thereby employing path analysis. All effects were controlled in terms of gender, age, occupational status, nationality, discipline, and employment level.
The model showed a marginal fit Y-B X2(253) = 641.21, p <0.001; SRMR = 0.09; CFI = 0.89; RMSEA = 0.06 (90% CI of 0.056, 0.067). The examination of the indices of modification (MI) revealed the addition of entrepreneurial engagement as an independent variable to further explain PBC (MI = 84.56), SN (MI = 40.07) and ATT (MI = 16.56). The fit of the final re-specified model was acceptable, Y-B X2(299) = 522.09, p <0.001; SRMR = 0.06; CFI = 0.95; RMSEA = 0.04 (90% CI of 0.037, 0.049), indicating the measurement of the latent variables as sound [67, 76]. The model explained 45.8% of the variance of EI among respondents. The results for the SEM are depicted in Fig. (1).
In hypothesis (H1a), we expected that entrepreneurial engagement would predict EI, beyond the effect of the TPB variables. In accordance with prior research, the three TPB-antecedents, ATT (β = 0.37, p < 0.001), SN (β = 0.12, p < 0.05), and PBC (β = 0.33, p < 0.001), were positively associated with EI. ENG exerted a positive influence on EI (β = 0.18, p < 0.001). Results provided support to the hypothesis (H1a) that there was a unique main effect of entrepreneurial engagement when predicting EI.
We also found evidence of a direct impact of ENG on TPB-antecedents. More specifically, the effect of engagement on PBC (β = 0.44, p < 0.001), on ATT (β = 0.16, p < 0.001), and on SN (β = 0.31, p < 0.001). Among the control variables, age (β = -0.11, p = 0.06), gender (β = 0.04, p = 0.39), employment level (β = -0.07, p = 0.18), discipline (β = 0.03, p = 0.55), and nationality (β = -0.09, p = 0.83) were not significantly related to EI.
5.2. Segmented Regression to Estimate the EI-growth Breakpoint
In hypothesis (H1a), we expected EI to increase during the entrepreneurial process, and for hypothesis (H1b), EI-growth to have a breakpoint per the context of a Rubicon crossing. First, an OLS regression revealed a positive effect of b0 = 0.23 (p < 0.001) on EI (R2 = 0.12). To create the scale of EI, values consisting of three items were aggregated. The piecewise regression estimated a breakpoint at 1.05 (St. Err = 0.56), suggesting two different segments of EI-growth. This breakpoint was statistically significant according to the Davis test for a change in the slope (p < 0.05; 95% CI = 0.04, 2.15). The next step was to perform an adjusted OLS regression, which revealed that the effect of engagement on EI in the first segment (Engagement < Breakpoint, b = 0.82, p < 0.001) differed compared to the second segment (Engagement > 1 Breakpoint b2 = 0.20, p < 0.001). The results showed that the growth of EI was stronger before an initial entrepreneurial action was taken and continued to grow at a lower rate. The results supported hypothesis (H1b) that a breakpoint in intention growth was visible at that moment respondents engaged in implementation (Fig. 2).
5.3. Moderating effects of entrepreneurial engagement within TPB framework
In hypothesis (H1c), we postulated a moderating effect of entrepreneurial engagement on the relationship between TPB-antecedents and entrepreneurial intentions (the criterion). A series of mean difference t-tests were performed, using the mean values of the manifest variables of each scale to create the variables. The two groups (pre-breakpoint vs. post-breakpoint) differed in PBC (t [432.2] = 10.1, p < 0.001, d=0.89), ATT (t [444.6] = 5.77, p < 0.001, d= 0.56), SN (t [465.8] = 8.54, p < 0.001, d= 0.74), EI (t [485.3] = 9.71, p < 0.001, d =0.84), and university spin-off rewards (t [505.2] = 2.53, p < 0.001, d= 0.21). The respondents in the group pre-breakpoint had a higher TPB-values (MPBC = 3.67, SDPBC = 1.54; MATT = 3.59, SDATT = 1.13; MSN = 2.80, SDSN = 1.52) compared to individuals in the post-breakpoint group (MPBC = 4.89, SDPBC = 1.26 MATT = 4.11, SDATT = 1.13; MSN = 3.86, SDSN = 1.40).
With regard to the control variables, we found group differences in age (t[517.31] = 3.5, p < 0.001, d=0.30), occupational status (Professor: No/Yes, ; χ2 [1] = 4.33, p <0.001; scientific assistants: No/Yes, ; χ2 [1] = 7.18, p<0.01); and gender (χ2 [1] = 17.87, p< 0.001) between the groups. Respondents in the pre-breakpoint group were slightly younger (M = 42.6, SD = 10.3) than the post-breakpoint group (M = 45.7, SD = 10.8). Additionally, in the post-breakpoint group, respondents were more likely to be male and professor. However, we did not find group differences in terms of employment level (t [530.28] = 1.16, n.s.), discipline (χ2 [1] = 1.13, n.s.) or nationality (χ2 [1] = 0.01, n.s.).
The results of the multi-group model show that in both groups, a different magnitude of EI variance was explained by the model (27.9% and 44.2%). Per our expectations, the TPB- antecedents positively explained EI (PBC: β = 0.19, p < 0.05; ATT: β = 0.36, p < 0.001; SN: β = 0.17, p < 0.01;) within the pre-breakpoint group. In the post-breakpoint group, the intention was significantly explained by ATT (β = 0.41, p < 0.001) and PBC (β = 0.39, p < 0.001), but not by SN (β = -0.01, p < 0.92). The results indicate that in the post-breakpoint group, the TPB antecedents PBC and ATT gain influence on EI.
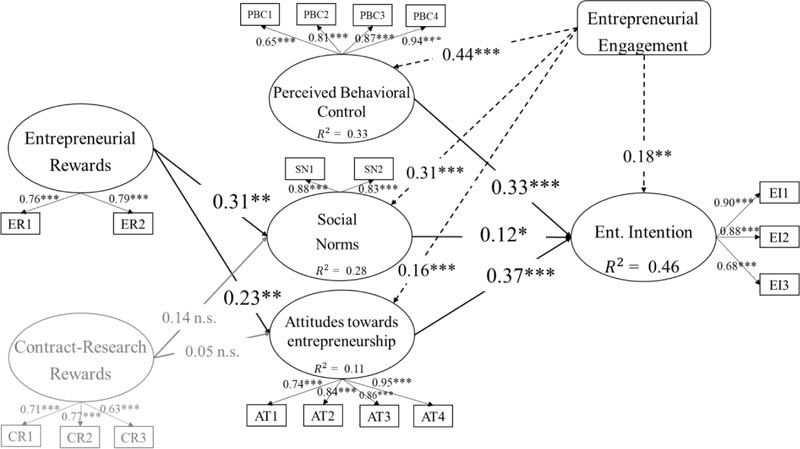
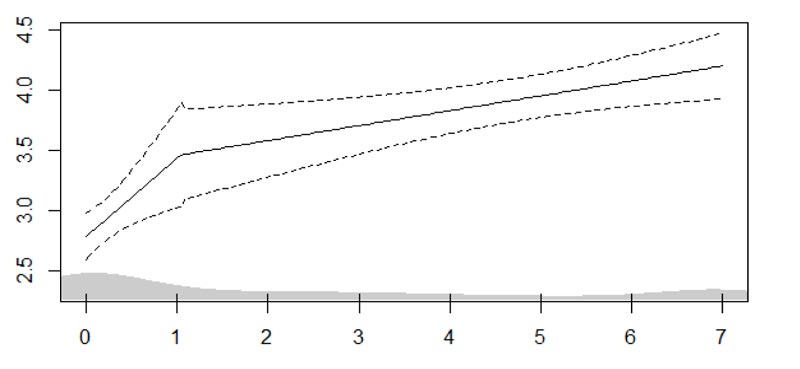
Models | X2 | df | CFI | RMSEA | ∆SBS-X2 | ∆dƒ |
---|---|---|---|---|---|---|
Unconstrained model | 621.77 | 426 | 0.929 | 0.047 | ||
ATT→EI set equal across groups | 626.25 | 427 | 0.927 | 0.048 | 5.904* | 1 |
PBC→EI set equal across groups | 634.85 | 427 | 0.924 | 0.049 | 12.824*** | 1 |
SN→EI set equal across groups | 623.67 | 427 | 0.929 | 0.047 | 1.412 n.s. | 1 |
ER→ SN set equal across groups | 622.22 | 427 | 0.929 | 0.047 | 0.383 n.s. | 1 |
ER→ ATT set equal across groups | 622.53 | 427 | 0.929 | 0.047 | 0.758 n.s. | 1 |
Next, we tested whether the group differences between the paths of TPB-variables and EI were statistically significant. We first tested a model that introduced no equality constraints as a function of order. This unconstrained model was tested against a model in which all factor loadings and all regression paths and covariance between latent variables were constrained to be equal across groups. Imposing equality constraints on the regression paths and covariance did not cause a significant reduction in model fit (∆SBS-X2(18) = 23.78, n.s) suggesting measurement invariance across both groups. Per the hypothesis (H1c), we tested the unconstrained model using three models in which one of the three paths of TPB variables to intentions was always set equal across both groups (see Table 5). We found evidence of a moderation effect in the case of the ATT-EI path and the PBC-EI path (as indicated by the significant (∆SBS-X2) thus, the hypothesis (H1c) was partially supported.
5.4. University Entrepreneurial Rewards
In hypothesis (H2a-b), we expected an indirect effect of the university spin-off rewards on EI, mediated by ATT and SN. The SEM depicted in Fig. (1) displayed a positive effect of spin-off rewards on SN (β = 0.31, p < 0.001) and ATT (β = 0.23, p < 0.001). Our additional control variable, the reward for commercialization for conducting contract research, had no positive effect on SN (β = 0.15, p < 0.07) or ATT (β = 0.01, p < 0.90). Thus, only university spin-off rewards and not contract-research rewards met essential criteria to serve as mediators [83] and were used for the following mediation analysis.
Regarding the mediation analysis, the overall model for SN and ATT as mediators between spin-off rewards and EI. The mediation hypothesis was supported by a significant indirect effect via ATT and SN on intention (βATT= 0.10; p < 0.001), which showed a possible range between CI0.95 = 0.07, 0.17, and via SN on intention (βSN= 0.07; p < 0.001) showing a possible range between CI0.95 = 0.04, 0.13 (R2TotalMed = 0.27). SN and ATT mediated the relationship between university spin-off rewards and EI (βTotal= 0.32; p < 0.001, βDirect= 0.15; p < 0.01), providing support for the hypothesis (H2a-b).
In hypothesis (H2c-d), we expected a moderating effect of entrepreneurial engagement on the relationship between spin-off rewards and AT and SN. To test the hypotheses, the multi-group SEM analysis, as previously presented in Table 5 was used. Therefore, we tested the unconstrained model using two models in which one of the reward paths SN and ATT was, in both cases, set equal across both groups. We found no evidence of a moderation effect in either the ER-ATT path or the ER-SN path.
6. DISCUSSION
This article is an initial step towards evaluating the influence of entrepreneurial engagement on the cognitive processes of potential entrepreneurs in the context of the TPB. The present study intended to uncover the effects of entrepreneurial engagement in the formation of entrepreneurial (goal-) intention. While this study sought to join together the broader research domain towards overcoming the differentiation of intention-based and action-based entrepreneurship research - more importantly, it responded to calls in the entrepreneurship literature. For example, Fayolle et al. [9], proposed a rethinking of the theoretical and conceptual use of intention in the phase preceding the launch of a new business. The present study builds on MAP to explain the influence of motivational factors on entrepreneurial intention. We investigated entrepreneurial decision-making in the academic field using a sample of Swiss scientists from various applied disciplines.
The concept of EI is an important instrument for investigating entrepreneurial conditions in the phase preceding the launch of a new business that favors subsequent entrepreneurial behavior. This study began to elucidate the deeper cognitive mechanisms that go beyond the predictors of the theory of planned behavior by adopting an action perspective. Building on the TPB and MAP models, we theorized a positive effect of entrepreneurial engagement, which extended beyond the antecedents of TPB (e.g., attitudes, perceived behavioral control, and social norms) to explain entrepreneurial intention. Furthermore, we investigated whether EI increased during the entrepreneurial process or whether the growth for EI peaked per context of a Rubicon crossing. Due to a changing cognitive mindset when moving into implementation, we investigated whether entrepreneurial engagement moderates the relationship between TPB predictors and entrepreneurial intention.
Briefly, the results of our empirical analyses demonstrated that ENG positively impacts EI beyond the TPB-antecedents. However, a segmented regression revealed that EI-growth significantly changed after implementing the first gestation action, suggesting the existence of an entrepreneurial Rubicon like demarcation during the pre start up phase. Furthermore, we found supporting evidence (with the exception of SN) for our hypothesis that ENG moderates the relationship between TPB-antecedents and EI (the criterion); such that pre-Rubicon the relationship is weaker and post-Rubicon the relationship is stronger. However, no moderating effect between SN and EI was evident in our data. These findings in the first part of our study, constructed an improved picture of EI before progressing into part two of the study.
Since we drew on cross-sectional data from the field of academic entrepreneurship, we further investigated whether the effects of university entrepreneurial rewards on TPB- antecedents vary after the transition to an action phase. While our data supported evidence of a positive indirect effect of entrepreneurial university rewards mediated via social norms and attitudes on the EI, we found no further moderating effect pre-/post-Rubicon for the relationships between entrepreneurial rewards and social norms, as well as rewards and attitudes.
6.1. Implications and Further Research
For three decades, scholars centered on EI rather than entrepreneurial performance when examining the role of personal and environmental drivers and barriers of entrepreneurship [9, 27]. The same trend is evident in the emerging field of academic entrepreneurship [11, 25, 52, 84]. Implications of the current study are twofold - theoretical and practical. From a theoretical point of view, the conceptualization herein enlarged the existing body of intention-based entrepreneurship research [11, 84, 85].
Consistent with prior findings [11, 24], our results demonstrated that all TPB-antecedents positively predicted EI. When controlling for ENG, the TPB-construct ATT revealed the greatest effect on EI, which also agreed with a prior meta-analysis [21]. We hypothesized engagement as an additional predictor of EI. Our analysis revealed a unique primary effect of engagement on EI, beyond the TPB antecedents and control variables, as well as a significant positive effect of engagement on the TPB-antecedents. Our data confirmed that scientists engaged in entrepreneurial implementation exhibited higher values of EI, and higher values of ATT, PBC, and SN. Consistent with Mwangi and Rotich [28], ENG also contributed to the variance elucidation of the EI beyond the TPB antecedents and, thus, represents a meaningful predictor of intention.
Second, our study expands the intention-based literature by uncovering the cognitive mechanism during the transition from a motivational to an actional phase. The Rubicon model, based on MAP, postulates a change of the cognitive mindset, referred to as the crossing of the Rubicon when passing from a motivational to a volitional phase [3]. The crossing of the Rubicon relates to the fact that the gathered information positively contributed to the perceived desirability of an entrepreneurial behavior in the motivational phase such that individuals take initial gestation action for goal achievement. We hypothesized EI-growth to peak per the context of a Rubicon demarcation, or the point when individuals start to engage in implementation. The results of our segmented regression confirmed that EI-growth decreased after initiating the first gestation action. Although our analysis indicated that EI-growth during the motivation phase was higher than EI-growth after initiating gestation action, and despite being lower, it was still positively significant.
Scholars have noted that numerous barriers must be overcome and micro-tasks completed before entrepreneurial goals can be turned into an action [86], and that some people may stay for a long time in the so-called “still-trying phase” [87]. However, the long-term goal is constantly updated by carrying out micro-tasks for implementation. In turn, an increase in goal intention during the implementation phase is likely, as the person becomes more committed to their entrepreneurial goals by constantly updating the desirability and feasibility of the overlaying goals [37]. Frese [86] refers to the concept of loss aversion (i.e., the prospective theory [88], explained that individuals, after starting to invest, or in this case after performing a certain number of gestation actions, become tied to the achievement of goals. Our data showed a difference for the TPB-predictors before and after an EI-growth breakpoint. In particular, an increase in the values of the TPB-antecedents can be interpreted as a reaction to cognitive dissonance after decision-making (i.e., post-decision dissonance effect; [89]. Consequently, individuals are selective about new information and are more likely to focus on the information that supports their decisions retrospectively.
While our findings agree with the theoretical assumption of the MAP, they contrast with prior entrepreneurship research, which assumed that a few initial gestation actions were necessary to gather relevant information for decision-making in the motivational phase [1]. While we assumed, per the MAP, a Rubicon demarcation after the first gestation action, Delanoë Gueguen and Fayolle [1] found a Rubicon demarcation after three gestation actions. The reason for these divergences is caused by the deviating contexts and analytical methods to determine the Rubicon. Based on longitudinal data and moderation-analysis, Delanoë Gueguen and Fayolle [1] assumed that the entrepreneurial Rubicon demarcation is the moment when goal-intention loses the predictive power to explain behavior. However, per the MAP, our data showed that the Rubicon demarcation is likely to be the tipping point when individuals become involved in implementation behavior and thus biased proponents of their projects. We urge future research to test our operationalization through the application of longitudinal laboratory data in order to investigate cognitive mechanisms related to the demarcation of an entrepreneurial Rubicon.
Third, in response to the mixed, measured research of Delanoë Gueguen and Fayolle [1], we theorized that the motivational mechanisms described by TPB vary after the transition to implementation the author. They [1] conducted interviews with prospective and nascent entrepreneurs and found cognitive mechanisms in the motivation phase to differ from those in the implementation phase. In particular, the authors noted that in the motivation phase, difficulties were presented in an abstract and unspecific way, whereas in an action phase, participants reported more realistically about needs and current tasks.
We theorized an interactive effect of ENG and TPB-antecedents to emerge in the model. To prove the hypothesis, we drew on our prior findings (the demarcation of an entrepreneurial Rubicon) and constructed two groups (pre-and post-breakpoint of EI-growth). In contrast to the pre-Rubicon group, the post-Rubicon group contained all participants that reported being involved in implementation. SEM-multi-group analysis revealed a positive moderation effect of the TPB-intentions link. Across both groups, the “routes” toward the formation of EI were different. Notably, our moderation test showed that the effects of perceived behavioral control and attitudes on EI differed significantly between both groups. While the effect of SN on the EI was not moderated, PBC and ATT gained importance for predicting EI among participants in the post-Rubicon group.
Prior research found PBC to be a strong predictor of entrepreneurial performance [27]. In a recently published interview, Ajzen assumed that complex goals such as entrepreneurial activities have to be viewed from a micro-task perspective, which in turn requires implementation intention [90]. By identifying higher means of PBC in the advanced entrepreneurial process, our results confirm that the participants developed the necessary skills to initiate the implementation. These findings are in line with research from social psychology, which shows that participants in an implemental mindset exhibit a higher level of self-efficacy [39] and more risk-taking behavior [40]. Our data demonstrated that during the entrepreneurial process, ATT and PBC gained importance as a direct predictor of EI.
In the second part of our study, we drew on the institutional theory [10] to analyze the influence of environmental factors, namely entrepreneurial rewards on the TPB-antecedents, to indirectly predict EI. While prior research found a direct effect of university rewards on EI [13], we tested for a mediation effect using SN and ATT as mediators. The results indicated that entrepreneurial rewards indirectly affect EI. Then we investigated whether the effect of rewards on SN and ATT was moderated by entrepreneurial engagement. We argued that in the motivational phase, rewards serve as the initial inspiration for hypothetical behavior and, therefore, directly influenced EI. Our results are consistent with prior research [47], which who claimed that social prestige and financial rewards are important incentives to foster academic entrepreneurship.
6.2. The Influence of Entrepreneurial Engagement on Intention
In line with Krueger [91], our study intended to re-fuel the discussion concerning the importance of EI in entrepreneurship research. In entrepreneurship research, intentions are considered a major immediate antecedent of behavior. Entrepreneurship research [1, 5, 6] recently introduced MAP into the field to predict entrepreneurial action. The application of action-oriented approaches has several advantages.
First, it supports investigating the entire entrepreneurial process from intention-formation to implementation of actions. Various endogenous and exogenous determinants can be investigated against the background of the individual entrepreneurial process, and practical consequences can be derived more precisely. Therefore, the application of the MAP to study endogenous and exogenous determinants of entrepreneurial action presents an intriguing avenue for future research.
Second, MAP allows for a distinction to be made between a goal and implementation intentions, which leads to a precise prediction of future entrepreneurial behavior [6]; though this distinction regarding intentions is rarely applied in entrepreneurship research. A recently published longitudinal study demonstrated that implementation intention mediated the relationship between goal intention and actual performance. However, goal intention moderated the relationship of implementation intention on performance [6]. Implementation intention requires effective planning and concerns people following the enactment of goal directed behaviors [7]).
Moreover, strong effects of if-then plans related to implementation intention only emerge when participants hold strong respective goal intention [92], suggesting the importance of goal-intention in the pre-launch phase of a new business. Future research is needed to examine whether cognitive strategies differ in detail at critical moments within the entrepreneurial process, before and after engaging in implementation. Future research could also examine the role of team processes related to the formation of goal-and implementation intention.
Our study contributed to the existing intention-based research by emphasizing that engagement is a significant predictor of EI, as well as of the TPB-antecedents. Academic entrepreneurship research, which applied intention-based approaches, has focused almost exclusively on the notion of EI by referring to goal-intention (e.g., as part of the TPB), which directs the focus towards only the motivational aspects of EI, without considering whether participants are in the motivation or implementation phase. However, our results highlighted that the transition from a motivational to a volitional stage (i.e., the crossing of the Rubicon) is associated with a change in the cognitive mindset. More research is required to understand the needs related to cognitive mechanisms in the implementation phase among scientists. Scholars using the TPB [8] should critically rethink the role of EI when examining conditions for entrepreneurial behavior. Our study encourages scholars to consider whether participants are in the pre-decisional phase (e.g., aspiring entrepreneurs) or the actional phase (e.g., nascent entrepreneurs focused on implementation). Overall, we urge future research to follow the new trend in entrepreneurship to apply action-related approaches [93].
Our study has important practical implications for policymakers and entrepreneurs. For practitioners, the results highlighted that environmental conditions, namely spin-off rewards, positively influence the perception of desirability along the entrepreneurial process. In line with prior research [11, 16, 94], universities need to develop instruments to support entrepreneurial decision-making according to the individual founding process. Individual information processing and specific needs, in terms of support, are altered by the individual entrepreneurial progress.
7. LIMITATIONS AND RECOMMENDATIONS
We acknowledge that our study is not free of caveats and that the limitations of this study offer opportunities for future research. Firstly, cross-sectional approaches are subject to criticism since there are no controls for individual-level effects. Our results should be verified with longitudinal data to further investigate an entrepreneurial Rubicon, and estimate the non-linear threshold effect we postulate. Second, the current study sought to measure the status of entrepreneurial progress as the sum of gestation actions. The measurement of entrepreneurial engagement should be validated using different samples and contexts. Different gestation actions require different amounts of time and effort (e.g., informing friends and family about the project vs. developing a prototype) and, therefore, individual gestation actions are hardly comparable and cannot be aggregated. Furthermore, the content of gestation actions is hardly transferable to all areas of entrepreneurship. Future research must rely on measures that address this problem by not referring to specific actions. For example, by applying a 10-point-Likert-scale and asking: “If you are currently in the process of founding your own company, where would you place yourself or your team in this process?” and provides options such as 1: “Not existing/Start-Up idea” to 10: “The Company has already been founded.”
Third, more exogenous factors, such as formal factors per Kirby et al. [44], should be included in the analysis to contrast our analysis, considering entrepreneurial rewards. As formal factors tend to address concrete problems in the implementation phase, we would expect formal factors to be more important in the (pre)-action phase. Furthermore, our study has contextual limitations as our sample contained very few women and scientists from Swiss UASs. These individuals also have a very high level of entrepreneurial experience, which is not unusual for UAS in Switzerland, as previous research shows [55]. We urge future research to test different contexts, including cross-country and multi-discipline comparisons.
CONCLUSION
Using academic entrepreneurship as an intended career choice [47, 95], our insights highlighted the need for targeted and personalized support for spin-off creation. Universities should offer individual-adaptable support in the form of coaching and training. Therefore, this study supports the call to analyze more action-relevant factors when examining the framework conditions for venture creation [9]. Our results increase the available knowledge concerning entrepreneurship and support the need for future research to control for entrepreneurial engagement while addressing EI. However, to guide policymakers, much more research untangling the cognitive mechanisms behind the business implementation is required.
NOTES
1 For an overview of applications of the TPB in the field of academic entrepreneurship see the recently published reviews [16, 17].
2 Gestation action refers to the necessary action that are required to be undertaken to create a new venture. Some scholars use the actual number of gestation actions undertaken by individuals to determine whether they are actively involved in the development process of their venture [32]. Delanoë Gueguen and Fayolle [1] adapted the gestation action suggested by Reynolds [33] to predict a psychological Rubicon.
LIST OF ABBREVIATIONS
ATT | = Attitudes towards academic entrepreneurship |
EI | = Entrepreneurial Intentions |
ENG | = Entrepreneurial engagement |
ER | = Entrepreneurial rewards |
MAP | = Mindset theory of action phases |
PBC | = Perceived behavioral control |
TPB | = Theory of planned behavior |
SN | = Social norms |
SEM | = Structural equalization modeling |
STEM | = Natural and technical sciences |
UAS | = Universities of applied sciences |
ETHICS APPROVAL AND CONSENT TO PARTICIPATE
Ethics approval was not required for this type of research, which was founded by the Swiss National Science Foundation. We used cross-sectional data and asked participants to participate in the study according to the European Union Data Protection Directive. However, no animals and only anonymous data of participants are involved in my study.
HUMAN AND ANIMAL RIGHTS
Not Applicable.
CONSENT FOR PUBLICATION
Informed consent was obtained from all the participants when they were enrolled.
AVAILABILITY OF DATA AND MATERIALS
The data that support the findings of this study are available from the corresponding author [R.B] upon request.
FUNDING
Financial support provided by the Swiss National Science Foundation (SNSF) in undertaking this research (ID 10001A_172554), Switzerland.
CONFLICT OF INTEREST
The authors declare no conflict of interest, financial or otherwise.
ACKNOWLEDGEMENTS
The authors gratefully acknowledge the financial support provided by the Swiss National Science Foundation (SNSF) in undertaking this research (ID 10001A_172554), Switzerland.